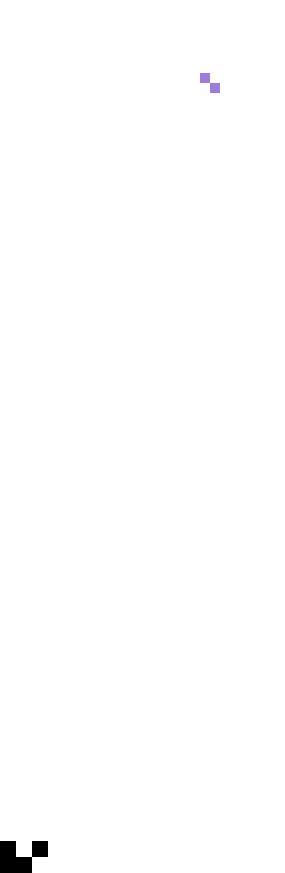

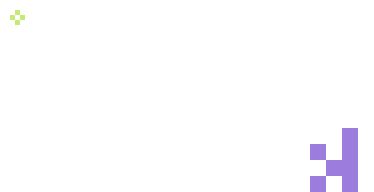
26/07/2024
Find out why User Testing is crucial for effective data visualisation. Find out how to optimise your graphs and dashboards through real tests with users, guaranteeing an intuitive and precise experience.
In the process of designing analytical applications with data visualisations, user testing is an essential step when it comes to understanding how users interact with various graphs, scorecards or visual elements that make up a given tool. In this article, we will explore in detail user testing, the most advanced techniques within our reach and the insights that this type of test can give us in the process of improving and evolving our visualisations.
Essential User Testing: Key to Success in UX/UI Design
In a world awash with details, where information is abundant but understanding is scant, data visualisation tools act as bridges between raw numbers and human understanding. However, the effectiveness of these tools is not measured just by their capacity to show information, but also by how the users receive it, interact with it and, as a last resort, use it to take decisions based on metrics.
User testing, in this context, takes on critical importance. Studies like the one by Heer and Shneiderman (2012) underline the need to incorporate user testing into the process of designing data visualisations. This user-focused approach is used to identify areas of improvement and optimisation that might otherwise go unnoticed.
Beyond the visual: Measuring the Cognitive Load and how information is consumed
Although the direct observation of how the end consumer interacts with data is essential in user testing, its true value lies in the more detailed and complex measurements, which reveal internal aspects of the cognitive process. Here is where devices like the eye tracker come into play, along with devices for measuring biometric parameters (blood pressure, perspiration, heart rate, etc.).
What are eye trackers and how do they work?
Eye trackers, as explained in the study by Goldberg et al. (2019), are crucial devices for understanding how users explore data visualisations and the order in which they consume the information. By tracking eye movements, researchers can identify attention patterns and areas of interest, as well as invisible or irrelevant areas, which allows optimising the design for a faster and more effective understanding of the data.
What are biometric devices and how do they work?
Biometric devices offer an even more detailed perspective, capturing physiological responses that reflect the user’s cognitive load. Research, like the one by Picard et al. (2001), highlights how changes in heart rate and skin conductance can reveal emotions and stress levels when interacting with data visualisations. This information is invaluable to adjust the design and minimise the user’s cognitive fatigue.
The Spectrum of Measurements: Memorability, Usability and More
Beyond the cognitive load, user testing can measure a plethora of aspects that influence the effectiveness and usefulness of data visualisation tools. Memorability, for example, is crucial to guarantee that users remember how to interact with the visualisation in future sessions.
A memorable design not only facilitates continuous use, it also promotes the long-term adoption of the tool.
Again,the eye tracker reveals contrasting information consumption patterns that tell us if a particular display will be easily recognisable and memorisable, or if it will be easily forgotten. Research such as the study by Midway et al. (2020) underscores the importance of memorability (or capacity to recall a visualisation) in the effectiveness of data tools. By identifying interaction patterns that contribute to greater memorability, designers can create more impactful and long-lasting experiences for users.
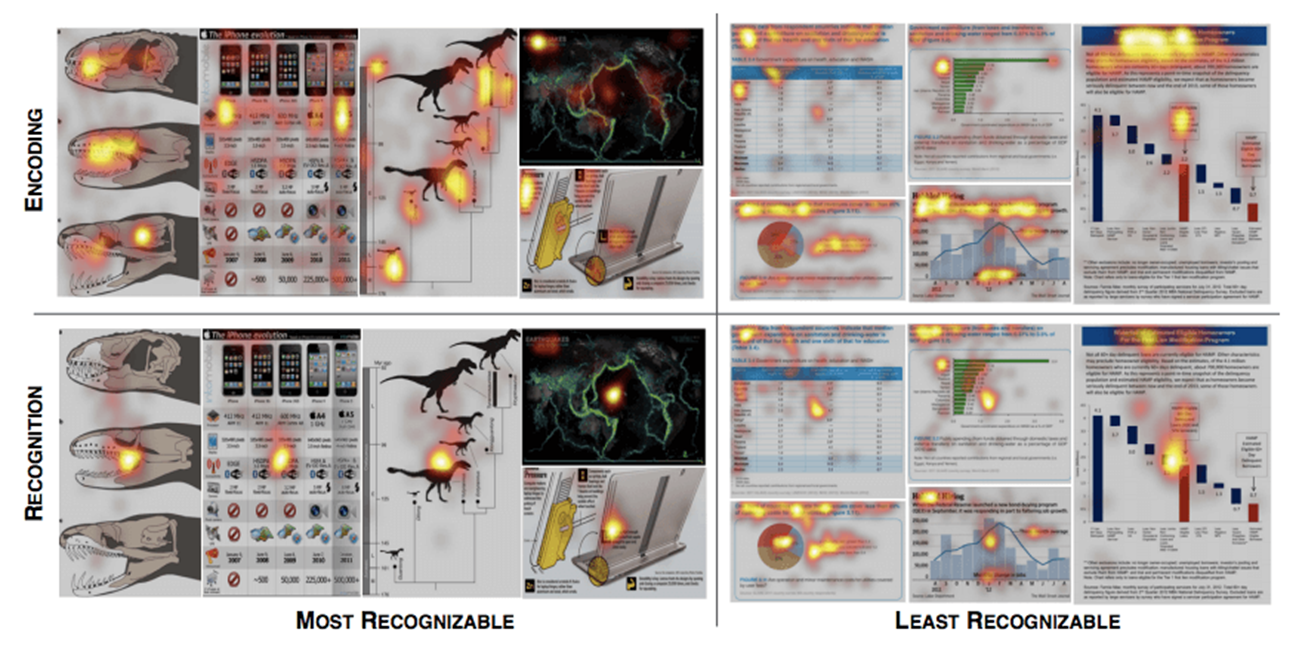
Image: Beyond Memorability: Visualization Recognition and Recall. 2015. The study reveals the differences in the consumption patterns of two visualisations. A) Recognisable: The second time that the user consumes the information, they recognise it clearly, specific pattern. B) The second time that the user consumes the information, they do not recognise the visualisation, scattered pattern very similar to the first time they consumed the information.
Usability is another key aspect that covers everything from ease of learning to the efficiency of use and user satisfaction. User testing can identify obstacles in the user workflow, areas of confusion and features that do not satisfy expectations. When dealing with these problems, designers can optimise the usability of the tool, improving the efficiency and reducing user frustration.
In addition, user testing can provide insights into the accessibility of the data visualisation, ensuring that it is useable by a wide range of users, including those with visual or cognitive disabilities. By making the visualisation more inclusive, its scope is expanded and it ensures that all users can benefit from the information it presents.
Validation Challenges and Data Visualisation Tests
Although user testing is essential to improve the user experience with data visualisations, other challenges must be addressed to yield accurate and significant results. These challenges include the selection of suitable participants and the interpretation of the data collected:
Selection of representative participants
One of the most significant challenges in user testing is ensuring that the participants are representative of the target audience. This can be difficult in data visualisations directed at specialised audiences or with very specific technical knowledge. The lack of representation can skew the results of the tests and lead to incorrect conclusions as to the effectiveness of the visualisation.
It is important that the participants be selected carefully, and that individuals are included who have a variety of backgrounds, skills and experience levels of relevance to the visualisation use case. It is also important to consider diversity in terms of age, gender, ethnicity and other demographic factors to ensure a broad and balanced perspective.
Design of realistic scenarios
Another common challenge in user testing is to create scenarios and tasks that precisely and realistically reflect the typical data visualisation use case. Scenarios that are too simplistic or unrealistic can underestimate the capabilities and needs of the user, while scenarios that are overly complex can overwhelm and distort the results of the test.
Designers must work closely with experts in the field and potential users to develop scenarios and tasks that are relevant, significant and realistic. This can entail the creation of specific use cases, the simulation of real-world situations and the incorporation of real or simulated data to provide an authentic context for the evaluation.
Interpretation of results and selection of metrics
Once the tests are completed, it is fundamental to interpret the results and define clear and significant metrics that can help us make improvements that will enhance our tool. The variety of data collected during the tests (completeness, times, pupillometry, number and duration of the fixations, in addition to the biometric data) can be overwhelming, and it is crucial to identify the most relevant and actionable findings to guide the design and development decisions.
Here is where performance and success metrics come into play. which can include task times, success rates, and more subjective metrics, such as user satisfaction and perceived usefulness. However, selecting suitable metrics can be a challenge in itself, since different stakeholders may have different priorities and success criteria.
Although user tests are essential to improve the user experience in data visualisations, they entail a series of unique challenges that must be addressed effectively and collaboratively. Similarly, managing the most significant findings identified will help us evolve and continuously improve data visualisations, to the benefit of users and organisations.
tags:
share: