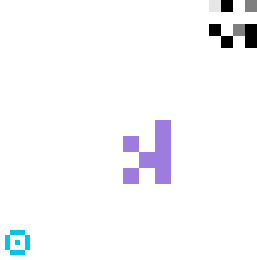

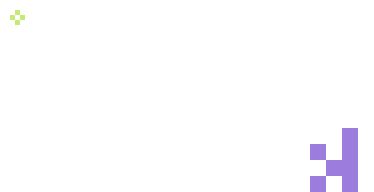
14/02/2024
Find out what Generative AI is, how it works and the primary ways in which it differs from traditional AI in this blog.
2023 has undoubtedly been the year of Generative Artificial Intelligence (GAI). Notable advancements have been made in this field, with continuous news surfacing throughout the year regarding the practical applications of generative AI in our daily routines, as well as the associated risks.
But do we know what Generative AI is and how it differs from traditional AI models?
Don’t miss the opportunity to enter the exciting world of Generative Artificial Intelligence (GAI)! If you want to discover how this innovative technology will transform our routine in 2024, read on!
Traditional AI vs. Generative AI. What are the main differences between them?
To comprehend the technological evolution, it is crucial to examine the differences between Traditional AI and Generative AI. Traditional AI operates based on predefined algorithms and historical data, enabling it to complete specific tasks. In contrast, Generative AI surpasses these capabilities by generating fresh content and adapting to novel situations with a creative approach.
Traditional AI models are based on algorithms that follow predefined rules and are designed to solve specific tasks, but cannot generalise or adapt to new contexts. In contrast, Generative Artificial Intelligence is a branch of artificial intelligence that focuses on the generation of (new and original) content, such as images, text, music, voice processing, video, etc. GAI uses machine learning techniques and neural networks to create models capable of generating content similar to that found in training datasets.
How does Generative Artificial Intelligence work?
Generative Artificial Intelligence operates in diverse ways, but it frequently relies on neural networks featuring Transformer architecture as a foundational element.
Transformer architecture: The purpose of Transformer architecture is to enable AI models to grasp meaningful connections among various components within the input data. For instance, in a text generation model, it facilitates understanding relationships between words in the input text and leveraging this understanding to generate an output.
A model like GPT chat, for example, “computes” the associations among words found in the given instructions. It generates text by combining the words that appear most probable based on this pattern of associations.
Together with the “Reinforcement Learning from Human Feedback”mechanism explained below, this architecture forms the two cornerstones that paved the way for the “Large Language Models” or LLMs as we know them today in 2024.
What are LLMs, what are they for and how do they work?
Large Language Models (LLMs) are artificial intelligence systems designed to understand and generate language by simulating human behaviour. These models are trained using sets of machine learning techniques, especially deep learning, on large amounts of data.
The operation of LLMs is based on neural networks and, in almost all cases, on “Transformers” architecture, such as the GPT-3 (Generative Pre-trained Transformer 3) model, developed by OpenAI.
These models are composed of multiple layers of processing units that interconnect and learn through millions or even billions of examples of text.
How are LLMs trained?
During training, LLMs are trained with large amounts of text, books, news articles, web pages and other resources available on the web. Using natural language processing and supervised learning techniques, the models learn patterns, relationships and language structures, as well as the ability to predict the next word given a context.
To use an LLM, a context or text input must be made available to it. The richer the context, the more accurate the model’s response will be. The model in turn generates an answer based on the input data and its prior knowledge acquired during training.
LLMs are statistical models and do not have a deep understanding of the meaning or context of the texts. Therefore, they can give very accurate answers in some cases, but they can also make mistakes and give wrong or inconsistent results. We refer to these answers as hallucinations. In addition, LLMs can also make errors due to inherent biases in the training data, which can affect the results generated.
Once an LLM has been trained, it can be used for a broad variety of language-related tasks. For example, it can complete sentences or texts, answer questions, translate texts from one language to another, produce coherent and relevant texts, summarise documents and much more.
But they can also give inappropriate responses, and different measures can (and should) be taken to ensure that these models are “Helpful-Honest-Harmless”.
RLHF for more accurate and honest answers
But how can we ensure that they are not dangerous and can be safely released to the public?
Another crucial breakthrough in the realm of generative AI is the reinforcement learning from human feedback (RLHF) mechanism. This advancement has facilitated the creation of models like GPT 3.5, which exhibit significantly enhanced reliability compared to their predecessors.
How does RLHF work?
To achieve this, the questions and answers produced by the LLM are presented to a panel of individuals who evaluate the quality of the answers. They determine if the responses adhere to the set rules or if they fall short. This feedback is then used to provide “rewards or penalties” to the model, enabling it to fine-tune its parameters through Reinforcement Learning.
Specifically, for the RLHF 3 guidelines are followed so that the results returned by the AI models align much more closely with what would be expected from a human agent:
- The LLM model is trained with pairs of prompts (i.e. “instructions” that the model must follow to generate an output) and their respective expected outputs.
- Then, for a large set of prompts, the LLM model is used to produce different outputs for the same prompt and a score is assigned to each output.
- Finally, the set of responses and points is used to train a “reinforcement learning“ model that biases the LLM model towards responses that would have received higher scores at point 2.
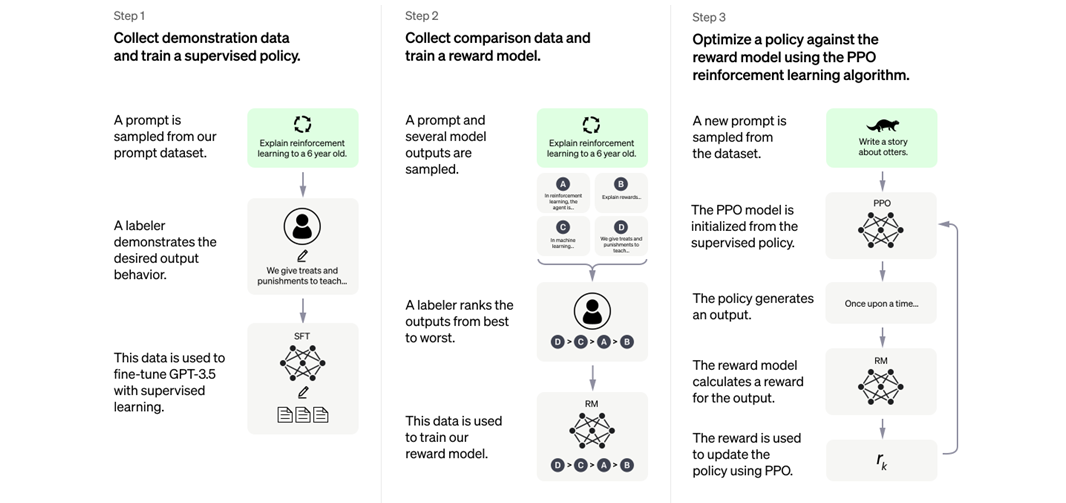
Diagram illustrating the three steps of the RLHF. Source: OpenAI
Comparative table on the most popular LLMs
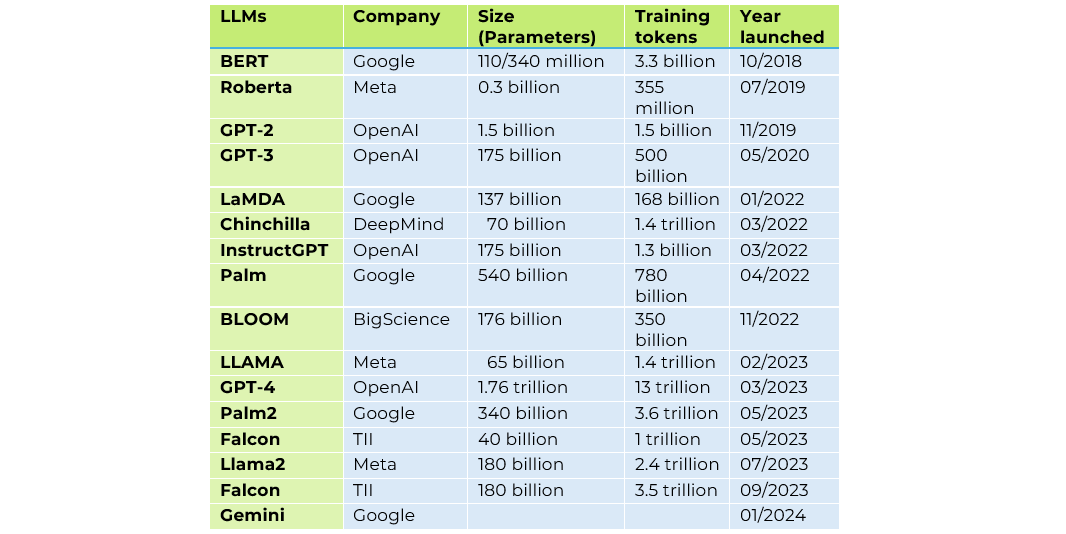
As we can see, the larger the models, the more capacities they have.
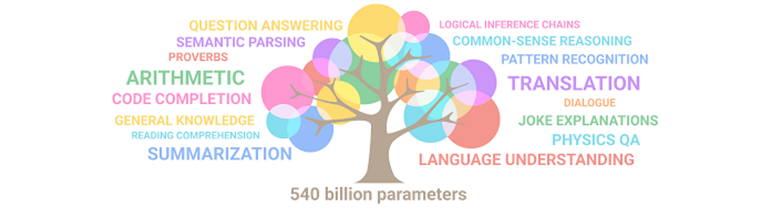
Illustration of how skills emerge in an LLM as it grows in size. Source: Google.
What capabilities can we find in LLM models?
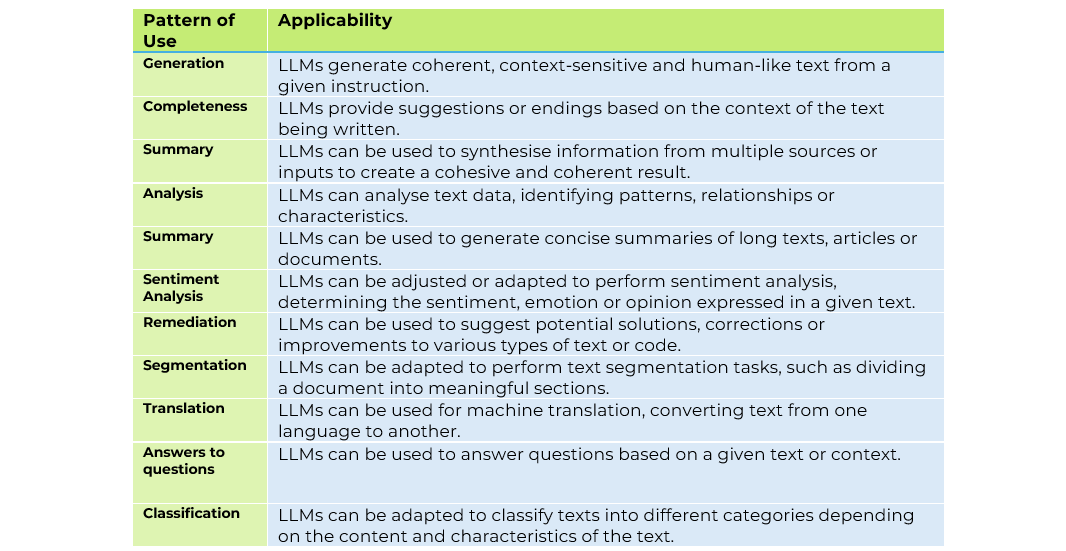
Driving generative Artificial Intelligence at CaixaBank with GenIAl
CaixaBank Tech actively participates in the GenIAl project, which has more than 100 professionals dedicated exclusively to the analysis and development of applications with generative Artificial Intelligence.
It is a multidisciplinary team that collaborates and adapts in a continuous learning environment. CaixaBank’s strategic partnership with Microsoft empowers us to co-create and access cutting-edge models and technological advancements that are not yet accessible in the market.
tags:
share: